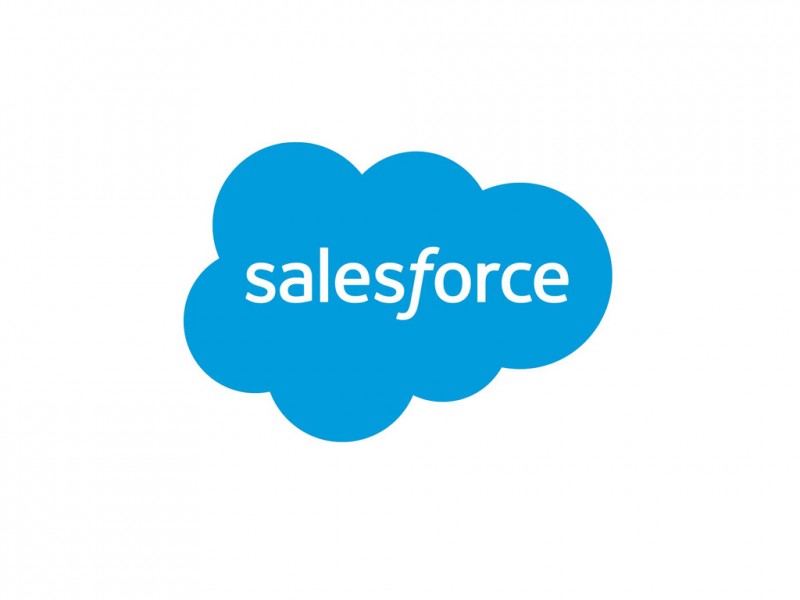
With 84% of CIOs believing AI will be as significant to business as the internet, strategic implementation is a competitive necessity. But many organizations aren’t sure where to start, how to scale AI agents, or how to measure success.
To provide a structured path for CIOs and IT leaders, Salesforce developed the Agentic Maturity Model, outlining four key stages of progression and actionable steps for advancement.
“While agents can be deployed quickly, scaling them effectively across the business requires a thoughtful, phased approach,” said Shibani Ahuja, SVP of Enterprise IT Strategy at Salesforce. “Understanding the progression of AI agent capabilities is crucial for long-term success, and this framework provides a clear roadmap to help organizations move toward higher levels of AI maturity.”
While agents can be deployed quickly, scaling them effectively across the business requires a thoughtful, phased approach.
Shibani Ahuja, SVP of Enterprise IT Strategy at Salesforce
This structured approach is already supporting companies investing in AI-driven automation, including:
- Wiley: “AI is complex and rapidly evolving, and a lot of leaders do not necessarily have clear visibility into where it’s going next,” said Kevin Quigley, Director of Process Improvement at Wiley, a multinational publisher of education materials. “As Wiley invests in agents, this model articulates how these solutions will come together as the technology matures and ensures that the building blocks we create today will set us up for success in the agentic future.”
- Alpine Intel: “As a business handling high volumes of insurance claims, every minute we save counts. Salesforce’s framework brings order to what can feel like chaos, offering a clear path for how and when to scale agentic capabilities,” said Kelly Bentubo, Director of Architecture at Alpine Intel, an insurance solutions provider. “It not only helps us identify where we are today, but sparks ideas for what’s next — from creating time-saving summaries to exploring multi-org applications across our acquisitions. That kind of clarity is rare in emerging tech, and exactly what we need to turn vision into value.”
The four levels of agentic maturity
Many organizations are still in the early stages of agentic AI adoption, having deployed chatbots and co-pilots. The next step is moving toward true agents that can operate autonomously and collaborate with humans. Here are the four levels of agentic maturity to guide that journey.
Level 0: Fixed Rules and Repetitive Tasks (Chatbots and Co-pilots)
What it is: Automation of repetitive tasks using predefined rules, with no reasoning or learning capabilities. At this level, chatbots and co-pilots are primarily focused on retrieving information (e.g., answering FAQs or handling basic inquiries). They help automate tasks, but do not recommend actions or take further steps. The shift to agentic AI begins when an agent moves beyond simply retrieving information to using reasoning to recommend or take action autonomously, as seen in higher levels of maturity.
Example: Chatbots handling basic service inquiries, like password resets or FAQ responses, following simple decision trees without adapting to new information
How companies can advance from Level 0 to Level 1:
- Identify use cases where chatbots and co-pilots are limited by rigid decision trees, and explore opportunities for reasoning capabilities to improve outcomes.
- Conduct time studies to quantify capacity saved by having agents automate tasks and recommend actions.
- Select use cases aligned with the organization’s risk tolerance and establish a mitigation strategy (e.g., Employee-facing Agent vs. Customer-facing Agent in regulated industries).
- Ensure data quality and availability by starting with a harmonized data source (e.g., one CRM Org) and plan for a broader data strategy as maturity increases.
- Focus not only on time savings but also on value and personalized customer experiences.
- Quantify time savings and demonstrate early efficiency metrics, such as reduced response times and cost savings from automation.
Level 1: Information Retrieval Agents
What it is: Agents that assist humans by retrieving information and recommending actions.
Example: An agent pulling data from a knowledge base to suggest the next steps for a customer support ticket, such as recommending relevant troubleshooting articles or escalating the issue.
How to advance from Level 1 to Level 2:
- Shift from recommendations to actions, allowing agents to autonomously execute tasks, reducing manual error and speeding up completion.
- Harmonize additional data, ensuring it is cleansed, transformed, and pre-processed as new sources are integrated.
- Establish a broader governance framework with testing methods, performance metrics, and continuous monitoring.
- Consider how user feedback will be gathered and incorporated.
- For customer-facing agents, ensure brand consistency is reflected.
- Measure ROI based on efficiency and effectiveness metrics like time savings, case deflection rates, and customer satisfaction.
Level 2: Simple Orchestration, Single Domain
What it is: Agents that autonomously orchestrate low complexity tasks in a siloed data environment.
Example: An agent that schedules meetings and automates follow-up emails using data from internal calendar and email systems.
How to advance from Level 2 to Level 3:
- Decide whether to build one multi-functional agent or multiple agents working together to achieve common goals. Consider latency impacts when choosing between a mega-agent or specialized agents.
- Grant agents access similar to human workers, ensuring separation of duties and applying the principle of least privilege.
- Design architecture to support future complexity, incorporating standards and protocols to avoid constant re-architecture.
- Use out-of-the-box API connectors to extend agents’ capabilities beyond single domains.
Level 3: Complex Orchestration, Multiple Domain
What it is: Agents that autonomously orchestrate multiple workflows with harmonized data across multiple domains.
Example: An agent handling complex cross-department workflows, such as managing the sales pipeline while pulling data from CRM, customer service tickets, and financial reports to create a holistic customer view.
How to advance from Level 3 to Level 4:
- Focus on use cases that require real-time, cross-domain agent collaboration, optimizing complex workflows with dynamic agent teams.
- Develop a universal agent communication layer (APIs, “agent bus”) for seamless collaboration.
- Implement dynamic agent discovery (registration, de-registration, and de-duplication) for ongoing maintenance.
- Design a scalable architecture that supports any-to-any agent interactions.
- Establish fine-grained access control, security policies, and a robust governance framework covering auditability, error handling, and transparency.
- Create layered human/AI supervision based on a risk framework.
- Develop a sustainable AI Agent Lifecycle Management methodology.
- Measure ROI by assessing efficiency, effectiveness, experience, and risk mitigation.
Level 4: Multi-Agent Orchestration
What it is: Any-to-any-agent operability across disparate stacks with agent supervision.
Example: Multiple agents from different systems collaborating to autonomously process orders, manage inventory, and route customer feedback across various departments in real time.
How to maximize value:
- Evolve security and governance for ecosystem-wide collaboration
- Explore new business models enabled by agent collaboration
- Develop metrics to quantify multi-agent system value
- Create feedback mechanisms to improve agent interactions
- Measure ROI by tracking overall business impact from multi-agent collaboration (e.g., revenue growth, cost savings, customer retention)
Beyond the technology: Critical considerations for agent implementation
“Organizations must look beyond the technology and consider the broader organizational impact,” said Ahuja. “This includes data readiness, security, and the need for a collaborative human-agent workforce.”
AI agents require more than just technical implementation — they demand a strong foundation in strategy, data, security, and workforce readiness. Organizations must align AI initiatives with business goals, ensure high-quality and integrated data sources, establish governance frameworks as agent autonomy increases, and invest in upskilling teams to foster seamless human-agent collaboration. Addressing these pillars accelerates adoption and drives sustainable AI maturity.
Learn More:
- Read more about agents through the launch of Agentforce 2dx
- Dive deeper into Salesforce’s CIO research
- See Agentforce in action with Alpine Intel
- Here’s how 1-800Accountant is scaling its expertise during tax season with Agentforce
Blog Article: Here