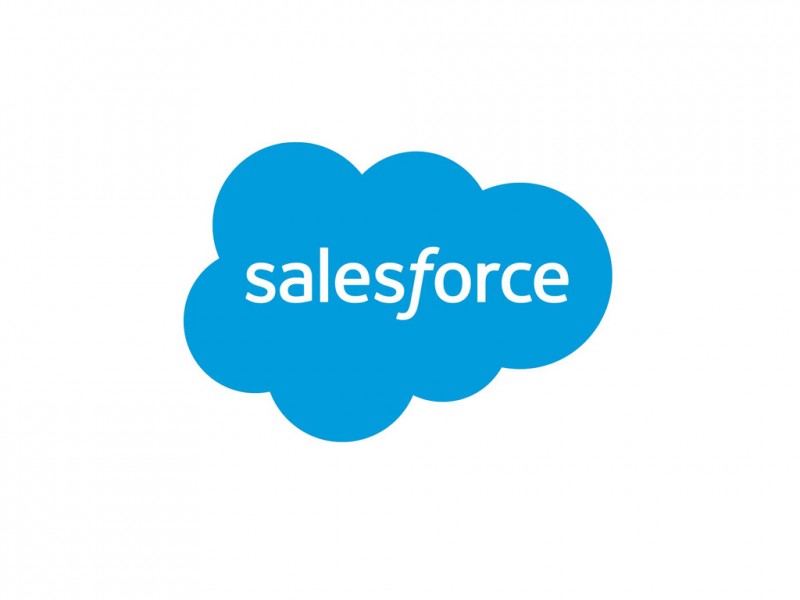
Every AI agent needs reasoning abilities. It’s what gives them the ability to analyze, adapt, and make meaningful decisions. But until Salesforce’s Agentforce came along, no AI agents, copilots, or assistants had a true, enterprise-class reasoning engine for transforming raw data and metadata into intelligent actions that mimic or even surpass human thinking.
“Reasoning engines combine models, data, business logic, events, and workflows into unified cognitive architectures,” said Phil Mui, Ph.D., SVP of Technology, Head of Products and Engineering, Salesforce AI Research and Agentforce. “Reasoning engines are compound systems. The good ones are fast inference time System 2 reasoners. They try to understand the nuances of user queries, contexts and provide accurate, faithful, and sometimes actionable responses.”
Reasoning engines combine models, data, business logic, events, and workflows into unified cognitive architectures.
Phil Mui, Ph.D., SVP of Technology, Head of Products and Engineering for Salesforce AI Research
This is a striking shift from the AI assistants we’ve come to know, with their scripted prompts and unpredictable replies. Inspired by noted psychologist Daniel Kahneman’s bestseller, Thinking, Fast and Slow, reasoning engines integrate the dual-process framework of human thought — the idea that we can sometimes be quick to react in a moment because a situation calls for it. Or we can take a moment, think about things more deeply, and come up with more intricate solutions to problems or needs.
In AI terms, many do-it-yourself (DIY) assistants, which are essentially thin software veneers over large language models (LLMs), go down that first rapid, or “System 1” path because they’re built for basic tasks like answering frequently asked questions or taking product orders. But organizations thinking about employing agents to offload or augment human work need higher levels of reasoning. This is where “System 2” inference comes into play with its ability to understand and think through a situation, deliver relevant insights, and suggest actions.
From quick fixes to thoughtful solutions
That’s part of what makes Agentforce — the first digital labor platform for enterprises — unique. By using System 2 inference-time reasoning, its Atlas Reasoning Engine, the “brain” behind Agentforce, can retrieve the most relevant data then reason and act, greatly improving processes across customer service, sales, and operations. When faced with a request, Atlas enables Agentforce to refine the query — expanding it with additional context — then performs advanced retrieval augmented generation (RAG) that pulls in relevant data and metadata while assessing the quality of its own response. This ability to answer a question, then reflect on the answer before seeking to answer again, enables more accurate responses and precise actions compared to other System 2 assistants that don’t have deep data and metadata context in Salesforce.
That step-by-step deliberate thinking also helps minimize one of the most pervasive and worrying pitfalls of AI: hallucinations. Indeed, 78% of workers would stop using an AI agent if it produced incorrect responses, according to a new Salesforce study. While System 1’s fast, instinctive response times can handle simple queries, it often falters in complex or ambiguous situations, leaving room for LLMs to fill in the blanks of its knowledge by delivering results that look real but are inaccurate, creating significant risks for businesses.
With hallucination rates for commercial AI chatbots ranging from 3% to as high as 27%, the value of deliberate, context-rich System 2 analysis is clear: Grounding decisions in deeper reasoning reduces errors while providing more complete and nuanced responses to complex questions. AI agents using System 2 reasoning, fueled by solutions like Salesforce Data Cloud that unify and harmonize business and customer data, further minimize hallucination risks while delivering more contextual and actionable insights — including inline citations that show the sources that Agentforce consulted to craft its response.
“We all know LLMs can hallucinate when there are requests without restraint,” said Claire Cheng, Ph.D., VP of Machine Learning and Engineering for Salesforce AI. “But by accessing the business domain and customer data, agents can reason much better and understand the context of a request to deliver more personalized and relevant responses.”
Cheng offers an example: Imagine a banking customer asking an AI agent to explain how interest rate changes might affect their portfolio. This is a complex question. A typical RAG system or simple AI assistant would probably generate a rudimentary response based on publicly available knowledge articles. However, it wouldn’t be able to evaluate other highly pertinent considerations, such as how much money the customer has in their investment accounts or the bank’s guidance on how to identify which of those funds might be sensitive to interest rate changes.
Agents able to perform deeper levels of data retrieval and reasoning, on the other hand, could provide a more comprehensive, detailed, and well-researched response to advise that banking customer’s investment strategy and even suggest a few in-house financial advisory options to guide their decision-making.
For instance, when a reasoning engine analyzes a customer support query, it doesn’t just provide a single answer for the user’s query. It evaluates every retrieved text chunk, reranks for the most relevant chunks, then synthesizes the final response based only on those that are relevant to the user’s query. With enhanced query processing techniques, and leveraging metadata-enriched indices, Agentforce customers have been able to achieve 33% improvement over DIY AI solutions in answer accuracy as well as doubling response relevance, as seen in early pilots of Atlas, Cheng said.
Businesses find a reason
For most businesses, the choice between an AI copilot and agent should be a no-brainer, she said. If all you need is answers to common questions, like what a college intern or entry level employee would be able to provide, then traditional AI assistants or copilots are fine. But if you want a higher level of expertise, then you need agents powered by reasoning engines.
Numerous companies are already taking advantage of these reasoning abilities. For example, The Adecco Group, a global leader in talent solutions, is transforming recruiting with Agentforce, which serves as both a recruitment assistant and a candidate concierge. Working 24/7, Agentforce efficiently processes millions of resumes, matches candidates to highly curated opportunities, and proactively prequalifies them. It also helps candidates refine their resumes, streamlining the hiring process and maximizing their potential. reMarkable, a premium digital tablet company, is using Agentforce along with Salesforce’s B2B Commerce solutions to provide customer service and commerce capabilities. Agentforce will handle common inquiries, allowing human agents to focus on more complex needs and higher-impact customer interactions. And 1-800 Accountant, a leading small business accounting firm, is using Agentforce to manage common inquiries and expects to deflect 65% of its incoming service requests as a result.
Numerous companies are already taking advantage of these reasoning abilities.
Those are just some of the possibilities agents offer when anchored in System 2 reasoning and customer data. Indeed, by combining role-based agents, data integration, and workflow automation, businesses can reap the benefits of agentic technology in every function.
What’s next for reasoning engines?
As AI continues to evolve, reasoning engines will play a critical role in advancing the capabilities of autonomous agents. Mui predicts these systems will become even more proficient with capabilities that enable AI agents to function as domain-specific experts rather than general-purpose assistants.
Reasoning engines will evolve to address increasingly complex interactions. Agentforce 2.0, announced today, can handle a wider variety of interactions, including those with multiple layers that require deeper thought. For example, a straightforward question like “What’s my portfolio status?” will employ basic reasoning for rapid responses. Alternatively, a deeper question, such as, “What’s the right investment vehicle for my child’s college fund based on my income and risk preferences?” will employ enhanced reasoning with advanced data retrievers — improving the answer by refining the query before pulling both relevant data and context-specific metadata from Data Cloud.
“The difference between this new version of Agentforce and the previous one is that it’s now able to reason deeper, retrieve data with more advanced technology, and generate not just simple answers but actionable responses,” said Cheng.
The difference between this new version of Agentforce and the previous one is that it’s now able to reason deeper, retrieve data with more advanced technology, and generate not just simple answers but actionable responses.
Claire Cheng, VP of Machine Learning and Engineering for Salesforce AI
Future progress in multi-agent systems and collaborative AI could further expand the potential of reasoning engines. By coordinating specialized agents, businesses will be able to tackle complex challenges that require diverse expertise, from supply chain optimization to personalized healthcare. Before long, reasoning engines will also provide what’s needed for multiple agents to work together on various projects. A marketer launching a new product, for example, might employ a reasoning-powered agent to help build a campaign. The agent would collaborate with outbound agents to push out that campaign across relevant channels, engaging with customers as they have questions, then send to commerce-focused agents to complete the transaction.
For now, the leap from System 1 to System 2 reasoning represents a transformative moment in AI development. By integrating advanced reasoning engines, companies like Salesforce are paving the way for AI systems that are more accurate, reliable, and attuned to the complexities of real-world business needs.
“In the next few years, I’d expect we’ll be able to deliver more agentic experts,” Cheng said. “The reasoning engine should be one of the first factors enterprise organizations consider when comparing digital labor options.”
Go deeper:
- Learn more about the Atlas Reasoning Engine
- Read the latest Agentforce news
- Learn more about Agentforce and Data Cloud
- Check out Agentforce customer stories
Blog Article: Here