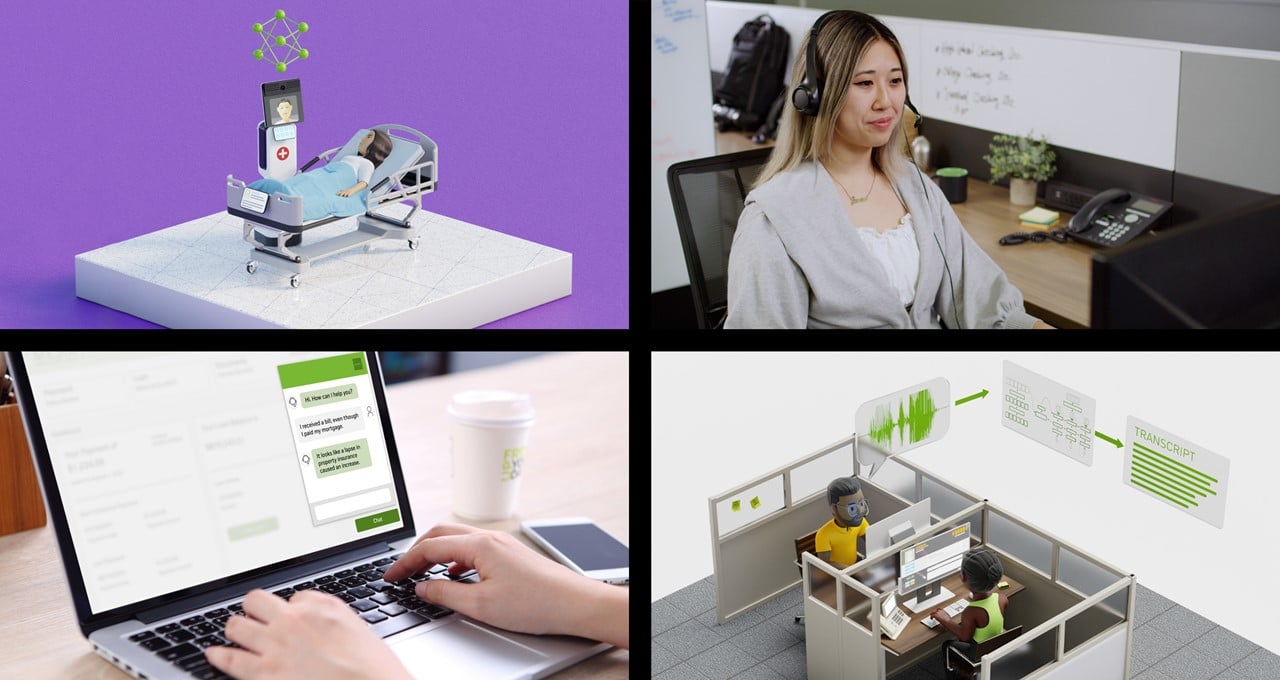
Customer service departments across industries are facing increased call volumes, high customer service agent turnover, talent shortages and shifting customer expectations.
Customers expect both self-help options and real-time, person-to-person support. These expectations for seamless, personalized experiences extend across digital communication channels, including live chat, text and social media.
Despite the rise of digital channels, many consumers still prefer picking up the phone for support, placing strain on call centers. As companies strive to enhance the quality of customer interactions, operational efficiency and costs remain a significant concern.
To address these challenges, businesses are deploying AI-powered customer service software to boost agent productivity, automate customer interactions and harvest insights to optimize operations.
In nearly every industry, AI systems can help improve service delivery and customer satisfaction. Retailers are using conversational AI to help manage omnichannel customer requests, telecommunications providers are enhancing network troubleshooting, financial institutions are automating routine banking tasks, and healthcare facilities are expanding their capacity for patient care.
What Are the Benefits of AI for Customer Service?
With strategic deployment of AI, enterprises can transform customer interactions through intuitive problem-solving to build greater operational efficiencies and elevate customer satisfaction.
By harnessing customer data from support interactions, documented FAQs and other enterprise resources, businesses can develop AI tools that tap into their organization’s unique collective knowledge and experiences to deliver personalized service, product recommendations and proactive support.
Customizable, open-source generative AI technologies such as large language models (LLMs), combined with natural language processing (NLP) and retrieval-augmented generation (RAG), are helping industries accelerate the rollout of use-case-specific customer service AI. According to McKinsey, over 80% of customer care executives are already investing in AI or planning to do so soon.
With cost-efficient, customized AI solutions, businesses are automating management of help-desk support tickets, creating more effective self-service tools and supporting their customer service agents with AI assistants. This can significantly reduce operational costs and improve the customer experience.
Developing Effective Customer Service AI
For satisfactory, real-time interactions, AI-powered customer service software must return accurate, fast and relevant responses. Some tricks of the trade include:
Open-source foundation models can fast-track AI development. Developers can flexibly adapt and enhance these pretrained machine learning models, and enterprises can use them to launch AI projects without the high costs of building models from scratch.
RAG frameworks connect foundation or general-purpose LLMs to proprietary knowledge bases and data sources, including inventory management and customer relationship management systems and customer service protocols. Integrating RAG into conversational chatbots, AI assistants and copilots tailors responses to the context of customer queries.
Human-in-the-loop processes remain crucial to both AI training and live deployments. After initial training of foundation models or LLMs, human reviewers should judge the AI’s responses and provide corrective feedback. This helps to guard against issues such as hallucination — where the model generates false or misleading information, and other errors including toxicity or off-topic responses. This type of human involvement ensures fairness, accuracy and security is fully considered during AI development.
Human participation is even more important for AI in production. When an AI is unable to adequately resolve a customer question, the program must be able to route the call to customer support teams. This collaborative approach between AI and human agents ensures that customer engagement is efficient and empathetic.
What’s the ROI of Customer Service AI?
The return on investment of customer service AI should be measured primarily based on efficiency gains and cost reductions. To quantify ROI, businesses can measure key indicators such as reduced response times, decreased operational costs of contact centers, improved customer satisfaction scores and revenue growth resulting from AI-enhanced services.
For instance, the cost of implementing an AI chatbot using open-source models can be compared with the expenses incurred by routing customer inquiries through traditional call centers. Establishing this baseline helps assess the financial impact of AI deployments on customer service operations.
To solidify understanding of ROI before scaling AI deployments, companies can consider a pilot period. For example, by redirecting 20% of call center traffic to AI solutions for one or two quarters and closely monitoring the outcomes, businesses can obtain concrete data on performance improvements and cost savings. This approach helps prove ROI and informs decisions for further investment.
Businesses across industries are using AI for customer service and measuring their success:
Retailers Reduce Call Center Load
Modern shoppers expect smooth, personalized and efficient shopping experiences, whether in store or on an e-commerce site. Customers of all generations continue prioritizing live human support, while also desiring the option to use different channels. But complex customer issues coming from a diverse customer base can make it difficult for support agents to quickly comprehend and resolve incoming requests.
To address these challenges, many retailers are turning to conversational AI and AI-based call routing. According to NVIDIA’s 2024 State of AI in Retail and CPG report, nearly 70% of retailers believe that AI has already boosted their annual revenue.
CP All, Thailand’s sole licensed operator for 7-Eleven convenience stores, has implemented conversational AI chatbots in its call centers, which rack up more than 250,000 calls per day. Training the bots presented unique challenges due to the complexities of the Thai language, which includes 21 consonants, 18 pure vowels, three diphthongs and five tones.
To manage this, CP All used NVIDIA NeMo, a framework designed for building, training and fine-tuning GPU-accelerated speech and natural language understanding models. With automatic speech recognition and NLP models powered by NVIDIA technologies, CP All’s chatbot achieved a 97% accuracy rate in understanding spoken Thai.
With the conversational chatbot handling a significant number of customer conversations, the call load on human agents was reduced by 60%. This allowed customer service teams to focus on more complex tasks. The chatbot also helped reduce wait times and provided quicker, more accurate responses, leading to higher customer satisfaction levels.
With AI-powered support experiences, retailers can enhance customer retention, strengthen brand loyalty and boost sales.
Telecommunications Providers Automate Network Troubleshooting
Telecommunications providers are challenged to address complex network issues while adhering to service-level agreements with end customers for network uptime. Maintaining network performance requires rapid troubleshooting of network devices, pinpointing root causes and resolving difficulties at network operations centers.
With its abilities to analyze vast amounts of data, troubleshoot network problems autonomously and execute numerous tasks simultaneously, generative AI is ideal for network operations centers. According to an IDC survey, 73% of global telcos have prioritized AI and machine learning investments for operational support as their top transformation initiative, underscoring the industry’s shift toward AI and advanced technologies.
Infosys, a leader in next-generation digital services and consulting, has built AI-driven solutions to help its telco partners overcome customer service challenges. Using NVIDIA NIM microservices and RAG, Infosys developed an AI chatbot to support network troubleshooting.
By offering quick access to essential, vendor-agnostic router commands for diagnostics and monitoring, the generative AI-powered chatbot significantly reduces network resolution times, enhancing overall customer support experiences.
To ensure accuracy and contextual responses, Infosys trained the generative AI solution on telecom device-specific manuals, training documents and troubleshooting guides. Using NVIDIA NeMo Retriever to query enterprise data, Infosys achieved 90% accuracy for its LLM output. By fine-tuning and deploying models with NVIDIA technologies, Infosys achieved a latency of 0.9 seconds, a 61% reduction compared with its baseline model. The RAG-enabled chatbot powered by NeMo Retriever also attained 92% accuracy, compared with the baseline model’s 85%.
With AI tools supporting network administrators, IT teams and customer service agents, telecom providers can more efficiently identify and resolve network issues.
Financial Services Institutions Pinpoint Fraud With Ease
While customers expect anytime, anywhere banking and support, financial services require a heightened level of data sensitivity. And unlike other industries that may include one-off purchases, banking is typically based on ongoing transactions and long-term customer relationships.
At the same time, user loyalty can be fleeting, with up to 80% of banking customers willing to switch institutions for a better experience. Financial institutions must continuously improve their support experiences and update their analyses of customer needs and preferences.
Many banks are turning to AI virtual assistants that can interact directly with customers to manage inquiries, execute transactions and escalate complex issues to human customer support agents. According to NVIDIA’s 2024 State of AI in Financial Services report, more than one-fourth of survey respondents are using AI to enhance customer experiences, and 34% are exploring the use of generative AI and LLMs for customer experience and engagement.
Bunq, a European digital bank with more than 2 million customers and 8 billion euros worth of deposits, is deploying generative AI to meet user needs. With proprietary LLMs, the company built Finn, a personal AI assistant available to both customers and bank employees. Finn can answer finance-related inquiries such as “How much did I spend on groceries last month?” or “What is the name of the Indian restaurant I ate at last week?”
Plus, with a human-in-the-loop process, Finn helps employees more quickly identify fraud. By collecting and analyzing data for compliance officers to review, bunq now identifies fraud in just three to seven minutes, down from 30 minutes without Finn.
By deploying AI tools that can use data to protect customer transactions, execute banking requests and act on customer feedback, financial institutions can serve customers at a higher level, building the trust and satisfaction necessary for long-term relationships.
Healthcare and Life Sciences Organizations Overcome Staffing Shortages
In healthcare, patients need quick access to medical expertise, precise and tailored treatment options, and empathetic interactions with healthcare professionals. But with the World Health Organization estimating a 10 million personnel shortage by 2030, access to quality care could be jeopardized.
AI-powered digital healthcare assistants are helping medical institutions do more with less. With LLMs trained on specialized medical corpuses, AI copilots can save physicians and nurses hours of daily work by helping with clinical note-taking, automating order-placing for prescriptions and lab tests, and following up with after-visit patient notes.
Multimodal AI that combines language and vision models can make healthcare settings safer by extracting insights and providing summaries of image data for patient monitoring. For example, such technology can alert staff of patient fall risks and other patient room hazards.
To support healthcare professionals, Hippocratic AI has trained a generative AI healthcare agent to perform low-risk, non-diagnostic routine tasks, like reminding patients of necessary appointment prep and following up after visits to make sure medication routines are being followed and no adverse side effects are being experienced.
Hippocratic AI trained its models on evidence-based medicine and completed rigorous testing with a large group of certified nurses and doctors. The constellation architecture of the solution comprises 20 models, one of which communicates with patients while the other 19 supervise its output. The complete system contains 1.7 trillion parameters.
The possibility of every doctor and patient having their own AI-powered digital healthcare assistant means reduced clinician burnout and higher-quality medical care.
Raising the Bar for Customer Experiences With AI
By integrating AI into customer service interactions, businesses can offer more personalized, efficient and prompt service, setting new standards for omnichannel support experiences across platforms. With AI virtual assistants that process vast amounts of data in seconds, enterprises can equip their support agents to deliver tailored responses to the complex needs of a diverse customer base.
To develop and deploy effective customer service AI, businesses can fine-tune AI models and deploy RAG solutions to meet diverse and specific needs.
NVIDIA offers a suite of tools and technologies to help enterprises get started with customer service AI.
NVIDIA NIM microservices, part of the NVIDIA AI Enterprise software platform, accelerate generative AI deployment and support various optimized AI models for seamless, scalable inference. NVIDIA NIM Agent Blueprints provide developers with packaged reference examples to build innovative solutions for customer service applications.
By taking advantage of AI development tools, enterprises can build accurate and high-speed AI applications to transform employee and customer experiences.
Learn more about improving customer service with generative AI.
Blog Article: Here